
Scattered Data: An Ongoing Challenge
Many retailers have transactional data stored independently from web logs, and CRM data as well. Such scattered data can prove to be a nightmare for them. Retailers should aim to view each customer as a single entity with a combination of traditional as well as non-traditional data such as social media behavior to generate valuable insights, detect probable frauds, generate effective marketing campaigns, and offer a robust customer service.
Retail supply chains are largely driven by numbers and analytics, and sellers have been slow in embracing real-time analytics and unstructured data sets due to their complexity. With machine learning and AI, studying data and deriving value from it is now swift and automated. Retailers can use a combination of structured and unstructured real-time data to generate forecasts for deriving augmented pricing strategies and effective inventory management.
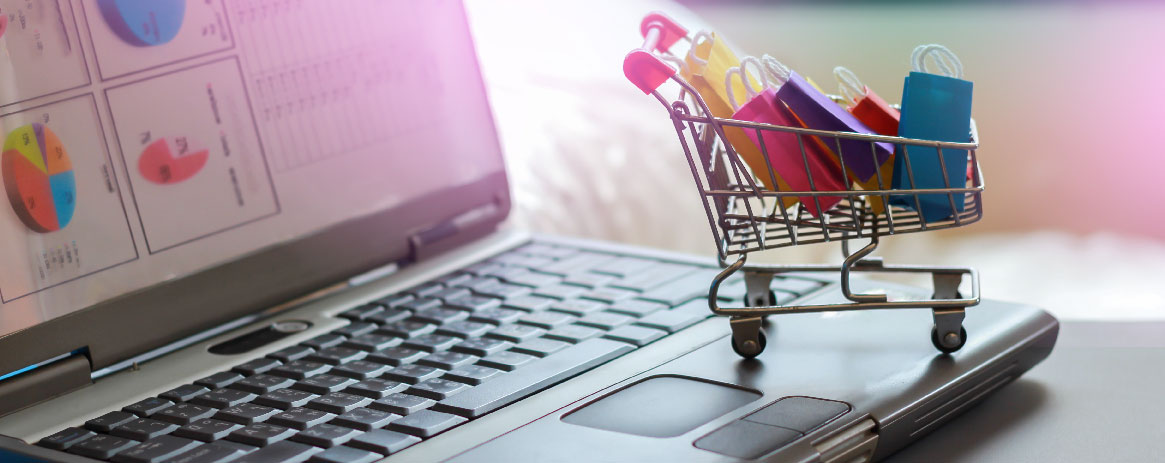
Avoid Mistakes Using Predictive Analytics
To study exhaustive amounts of data, retailers try to achieve valuable insights from every single metric of data available. This is bound to take them around in circles, without eliciting any real results. Instead, they should focus only on key metrics that are unique to their business goals.
Sticking to outdated statistical modeling is a common mistake as well. Creating static data models that only chart the hypothesis of a specific point in time should be avoided. It is advisable to create models that are updated automatically as principal pointers and key performance metrics. This helps in keeping real-time goals aligned to the company’s overall data analytics strategy.
DecisionMinesTM: Deriving Valuable Insights for Retailers
In this age of digital disruption, DecisionMinesTM combines the power of technology with innovative solutions. It offers an effective retail strategy by modelling all existing consumer data, with a deep focus on the organization’s ROI ambitions and brand acceptance. Trend prediction, workforce management, customer analytics, inventory management, and demand forecasting are some of the solutions offered by DecisionMinesTM.